Summary
Some of the systems that matter most to the Defense Department are very complicated.
Ecosystems, brains and economic and social systems have many parts and processes, but they are studied piecewise, and their literatures and data are fragmented, distributed and inconsistent. It is difficult to build complete, explanatory models of complicated systems, and so effects in these systems that are brought about by many interacting factors are poorly understood.
Big mechanisms are large, explanatory models of complicated systems in which interactions have important causal effects. The collection of big data is increasingly automated, but the creation of big mechanisms remains a human endeavor made increasingly difficult by the fragmentation and distribution of knowledge. To the extent that the construction of big mechanisms can be automated, it could change how science is done.
The Big Mechanism program aims to develop technology to read research abstracts and papers to extract pieces of causal mechanisms, assemble these pieces into more complete causal models, and reason over these models to produce explanations. The domain of the program is cancer biology with an emphasis on signaling pathways.
Although the domain of the Big Mechanism program is cancer biology, the overarching goal of the program is to develop technologies for a new kind of science in which research is integrated more or less immediately—automatically or semi-automatically—into causal, explanatory models of unprecedented completeness and consistency. Cancer pathways are just one example of causal, explanatory models.
The Big Mechanism program will require new research and the integration of several technical areas, particularly statistical and knowledge-based Natural Language Processing (NLP); curation and ontology; systems biology and mathematical biology; representation and reasoning; and quite possibly other areas such as visualization, simulation, and statistical foundations of very large causal networks.
Machine reading researchers will need to develop deeper semantics to represent the causal and often kinetic models described in research papers. Deductive inference and qualitative simulation will probably not be sufficient to model the complicated dynamics of signaling pathways and will need to be augmented or replaced by probabilistic and quantitative models. Classification and prediction will continue to be important, but causal explanation is primary. Extant databases and ontologies will provide top-down constraints on reading, assembly of big mechanisms and explanation.
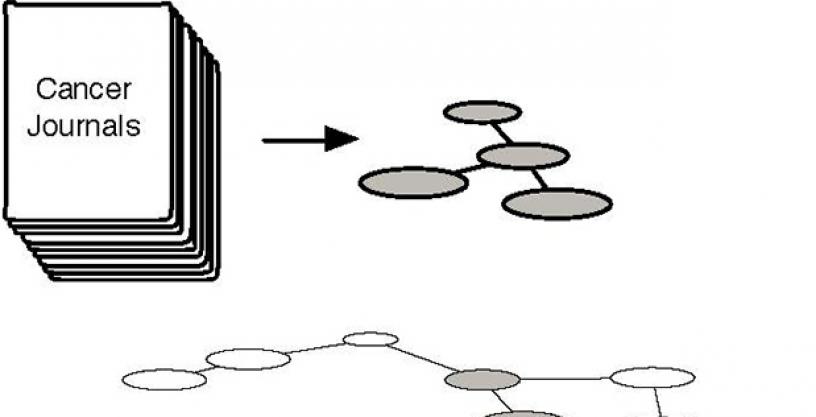
This program is now complete
This content is available for reference purposes. This page is no longer maintained.